FAIR Principle —Riding the Data Wave: Building a Sustainable Data Architecture
The amount of data we generate continues to explode at an unprecedented rate. Estimates suggest that in 2023, a staggering 120 zettabytes of data were created, captured, copied, and consumed — that’s a mind-boggling 120 trillion gigabytes in a single year! This exponential growth, coupled with the increasing speed and diversity of data, presents both challenges and opportunities for organizations.
In today’s data-driven world, having a well-defined data strategy is no longer optional, it’s essential for survival. With so much data being generated, organizations need a clear plan for what data to store, how to manage it, and how to leverage it for insights and competitive advantage.
A good data strategy helps organizations:
- Make informed decisions: By providing access to high-quality, reliable data, organizations can make data-driven decisions that are more likely to be successful.
- Optimize operations: Data can be used to identify inefficiencies, streamline processes, and improve overall operational efficiency.
- Enhance customer experience: By understanding customer data, organizations can personalize their offerings and interactions, leading to higher customer satisfaction and loyalty.
- Drive innovation: Data can be used to fuel innovation by identifying new opportunities, developing new products and services, and improving existing ones.
However, simply collecting and storing all available data is not only impractical but also counterproductive. It can lead to data silos, making it difficult to find and access the information needed, and incurring significant storage and management costs. A good data strategy helps organizations prioritize and curate their data, focusing on the information that is most valuable and relevant to their business objectives.
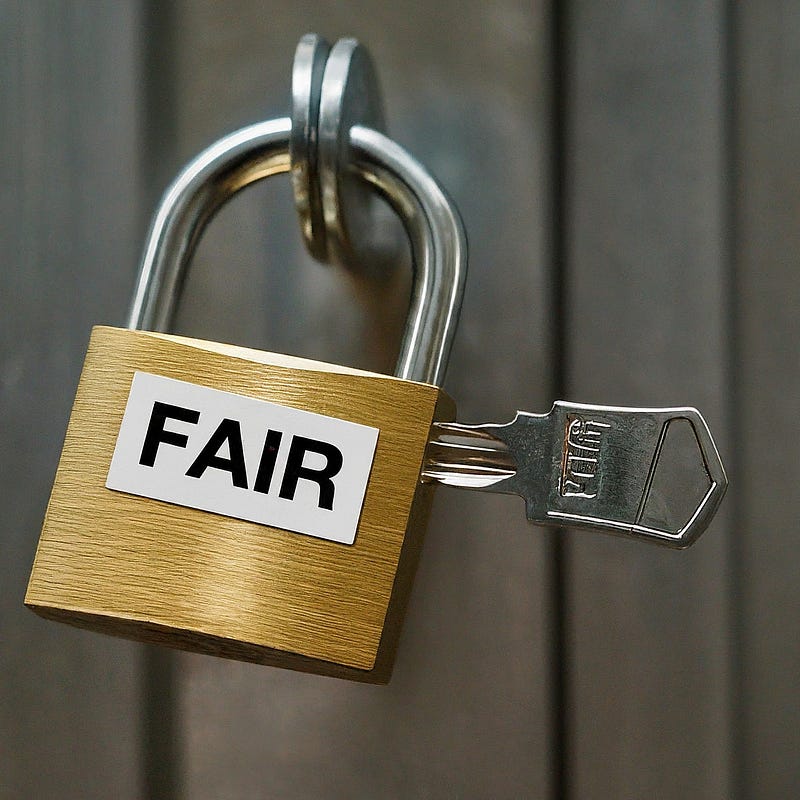
The Importance of FAIR Principles
The FAIR principles offer a blueprint for managing data in a way that ensures it is Findable, Accessible, Interoperable, and Reusable. Implementing these principles can significantly enhance the ability of organizations to derive value from their data.
Findable
- Rich Semantic Metadata: Ensure data is described using rich, semantic metadata to make it easily findable by both humans and computers.
- Persistent Identifiers (PIDs): Assign globally unique and persistent identifiers to both data and metadata to facilitate easy location and access.
- Data Catalogue: Index data and metadata in a searchable catalogue, ideally compliant with the Data Catalogue Vocabulary (DCAT), enhancing the discoverability of data assets.
Accessible
- Standard Data Protocols: Utilize standard data protocols (e.g., HTTP) for retrieving data and metadata using their PIDs, ensuring accessibility without the need for proprietary tools.
- Open Communication Protocols: Adoption of open communication protocols enhances the accessibility of data.
- Authentication and Authorization: Implement necessary security measures for data access while ensuring metadata remains accessible even if the data itself is not available.
Interoperable
- Common Formats and Vocabularies: Provide data in formats and vocabularies that are widely understood, preferably open formats, to facilitate interoperability.
- Knowledge Representation: Use controlled vocabularies and knowledge representation standards (e.g., RDF, OWL, SKOS) to define metadata, ensuring it includes qualified references to other metadata.
- Adherence to FAIR Principles: Ensure metadata vocabularies and standards follow FAIR principles to maximize interoperability.
Reusable
- Detailed Metadata Attributes: Equip metadata with detailed attributes to help users (both human and machine) ascertain the usefulness of the data.
- Data Usage License: Clearly define the data usage license to address legal interoperability and clarify user rights.
- Data Provenance: Record the data’s provenance, including its origin, any processing it has undergone, and any recompilations, to ensure transparency and trustworthiness.
- Industry or Community Standards: Align metadata with domain-relevant standards to ensure it meets the expectations and requirements of the specific industry or community.
Benefits of a FAIR Data Architecture
By embracing the FAIR principles, organizations can reap numerous benefits:
- Enhanced decision-making: Easier access to reliable data empowers data-driven decision-making across the organization.
- Improved collaboration: Sharing data becomes effortless, fostering collaboration and innovation within and beyond the organization.
- Reduced costs: Efficient data management lowers storage, retrieval, and analysis costs.
- Increased efficiency: Streamlined data processes save time and resources, allowing teams to focus on strategic initiatives.
- Future-proofed data infrastructure: A FAIR data architecture is adaptable and scalable, accommodating future growth and evolving needs.
Implementing a FAIR Data Architecture
There’s no one-size-fits-all approach, but here are some key steps to consider:
- Define your data strategy: Establish clear goals and objectives for data management.
- Identify data sources and requirements: Understand the data you collect, store, and utilize.
- Develop a data governance framework: Establish policies and procedures for data management.
- Invest in appropriate data tools and technologies: Choose tools that support FAIR principles and integrate seamlessly with your existing infrastructure.
- Foster a data-driven culture: Encourage data awareness and responsible data practices across the organization.
Conclusion
Building a robust data architecture in the Zettabyte Era requires a strategic approach grounded in the FAIR principles. By making data findable, accessible, interoperable, and reusable; organizations can not only manage the vast volumes of data generated daily but also unlock valuable insights and innovations. Implementing these principles effectively will position organizations to leverage their data assets fully, driving forward business intelligence, machine learning, and AI initiatives.
Leave a Reply