The Future with Enterprise Data Models in a Self-Serve World
Balancing Precision and Accessibility: The Journey from Data Chaos to Clarity
In the digital age, data is not just an asset; it’s the backbone of modern business strategies. As organizations grapple with vast volumes of data, the need for robust enterprise data models becomes more pronounced. These models are no longer luxuries but necessities, especially in a self-serve environment where access to reliable and consistent data is paramount.
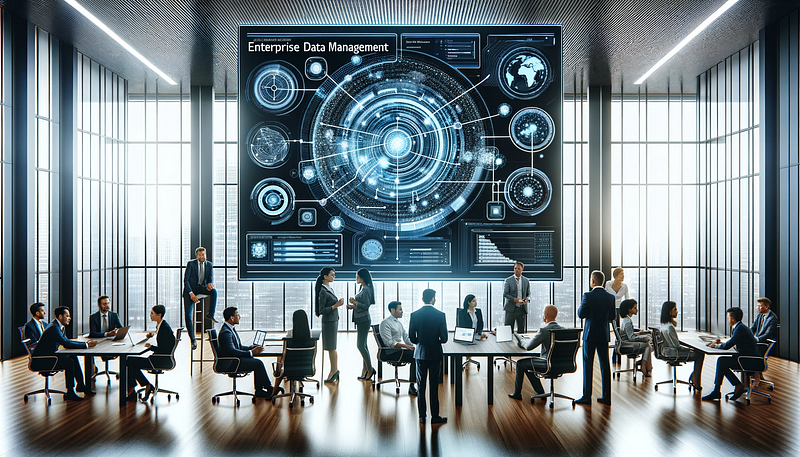
The Need for Clarity in Data Models
Ambiguity in data modeling is a major pitfall, leading to inconsistencies and confusion. The clear, standardized approach provided by enterprise data models is essential for maintaining data integrity across various business units.
Enterprise Data Models — A Pillar of Modern Business
In the realm of data, consistency, integration, quality, and governance are key. Enterprise data models serve as the backbone for these elements, providing a unified framework for data management and a standardized approach to data definitions, formats, and naming conventions.
- Defining Enterprise Data Models: An enterprise data model is an architectural blueprint that outlines how data is organized, managed, and utilized across an organization. It serves as a comprehensive guide for data collection, storage, access, and usage. This model includes the structure of the database, the relationships between different data elements, and the rules governing data interactions.
- Standardization and Consistency: One of the primary benefits of an enterprise data model is the standardization of data definitions and formats. In a large organization, different departments may use varying terms and formats for similar data. Enterprise data models bring uniformity, ensuring that everyone in the organization speaks the same data language. This consistency is crucial for accurate reporting, analysis, and decision-making.
- Data Quality and Integrity: These models play a vital role in maintaining the quality and integrity of data. By providing a structured approach to data management, they help in identifying and rectifying errors, inconsistencies, and duplications in data. High-quality data is essential for reliable analytics and business intelligence, which are key to informed decision-making.
- Facilitating Data Governance: Enterprise data models are integral to effective data governance. They provide a framework for data policies, standards, and practices, ensuring compliance with both internal policies and external regulations. This aspect is particularly important in industries like finance and healthcare, where data handling is subject to stringent regulatory requirements.
- Enhancing Data Accessibility and Utilization: In a self-serve data environment, where users across the organization need access to data for analysis and reporting, a well-defined data model simplifies data accessibility. It allows users to understand and utilize the data more effectively, fostering a culture of data-driven decision-making.
- Supporting Scalability and Flexibility: As businesses grow and evolve, their data needs change. Enterprise data models are designed to be scalable and flexible, accommodating new data sources, evolving business processes, and changing market demands. This scalability ensures that the data architecture can support the organization’s growth and adaptability.
- Integration of Diverse Data Sources: Modern businesses often deal with a range of data sources, including internal systems, cloud-based platforms, and external data feeds. Enterprise data models facilitate the integration of these diverse data sources, providing a unified view of data across the organization. This integration is key to comprehensive analytics and business intelligence.
- Enabling Advanced Analytics and AI: With the rise of advanced analytics and artificial intelligence, enterprise data models have become even more crucial. They provide the structured data foundation needed for machine learning algorithms and AI tools to function effectively, unlocking new insights and capabilities for businesses.
The Rise of Self-Service Data Models
Gone are the days when data management was the sole purview of IT departments. Today, we witness an increasing trend towards self-service models. These models empower individuals within organizations to directly access and analyze data, fostering a culture of agility and informed decision-making. However, this democratization of data brings its own set of challenges, primarily around maintaining data consistency and quality.
Traditionally, data management was largely centralized within IT departments. These departments were responsible for collecting, storing, managing, and distributing data across an organization. Access to data was restricted, and non-IT personnel had to rely on IT professionals for data reports and analysis, which could be time-consuming and often resulted in bottlenecks.
The Shift to Self-Service Data Models
1. Empowerment of Non-IT Staff: In self-service data models, the approach shifts significantly. These models enable employees across various departments to access and analyze data without direct IT intervention. Tools and platforms are designed to be user-friendly, allowing even those without technical expertise to derive insights from data.
2. Promoting Agility and Quick Decision-Making: This access facilitates agility in decision-making. Employees can quickly gather the data they need, analyze it, and make informed decisions. This speed is particularly crucial in dynamic market environments where delays in decision-making can lead to missed opportunities.
3. Encouraging a Data-Driven Culture: Such models promote a culture where data-driven decision-making becomes a norm. Employees are encouraged to base their strategies and day-to-day decisions on concrete data insights, leading to more effective and efficient business practices.
Self-Service vs. Curated Models
The dichotomy between self-service and curated models presents a strategic challenge for organizations. While self-service models offer direct access to data analysis, curated models prioritize data quality and standardization. Balancing these models is essential for agile and effective data governance.
Factors Influencing the Adoption of Enterprise Data Models
Several factors, including organizational size, data complexity, regulatory requirements, and decision-making needs, dictate the necessity for an enterprise data model. Tailoring the model to these factors ensures its effectiveness and relevance.
Cultivating a Data-Driven Culture
A robust data culture is integral to leveraging the full potential of enterprise data models. It involves nurturing data-driven decision-making, enhancing data literacy, and fostering innovation through data analysis.
Building and Scaling Enterprise Data Models: Key Considerations
The process of developing and expanding enterprise data models encompasses various considerations, including the role of data warehouses, the characteristics of a data model, the selection of appropriate tools, and balancing self-service with curated models.
From Personal Projects to Enterprise-Level Models
Many businesses evolve from personal projects with simple data structures to complex enterprise-level operations. This transition necessitates a scalable and performance-optimized data model to accommodate growing data demands.
Conclusion
Enterprise data models are pivotal in navigating the complexities of today’s data-driven business environment. They enable organizations to manage data effectively, ensuring consistency, reliability, and compliance with governance standards. By understanding and implementing these models, businesses can harness the power of their data to drive decision-making and innovation.
Leave a Reply